User:Farhan
Edge Computing Architecture and Layers[edit]
Introduction[edit]
Edge computing is a new model that locates computer processing near the source of data generation in an effort to restrict latency and bandwidth consumption. Edge computing is distinct from traditional cloud-based systems with centralized data centers employed for processing data in that computational power is spread across devices and local nodes within the network at the edge. The architectural change is a necessity in real-time applications with stringent high-speed data processing demands like autonomous cars, health monitoring, and industrial automation.
The infrastructure of edge computing consists of various layers, and each one performs its function. At the ground level, there are edge devices like sensors and actuators that collect data and perform the initial processing. Then there is fog computing, which is an intermediate layer between various edge nodes, collects data, pre-processes, and transmits it to the cloud. The upper cloud performs the heavy computation and long-term storage of data.
The confluence of IoT, mobile computing, digital twins, and cloud infrastructure creates a robust edge computing architecture. We present in this chapter the basic building blocks and layers of edge computing, such as IoT and digital twins, cloud infrastructure, fog computing, and the edge-cloud continuum.
2.1 IoT, Mobile, and Digital Twins[edit]
Internet of Things (IoT)[edit]
The Internet of Things (IoT) is a network of physical objects that interact via the internet. Examples range from common consumer devices such as smart thermostats, manufacturing equipment, health wearable sensors, and pieces of urban infrastructure. IoT provides additional connectivity and automation by way of ongoing data creation and analysis.
Key Features of IoT:[edit]
- Connectivity: Implements protocols like Wi-Fi, Bluetooth, Zigbee, and LoRaWAN for data transmission,
- Data Processing: Employs cloud and edge computing platforms to process the data that is gathered by sensors,
- Automation: Employs AI-driven or rule-based systems to make decisions autonomously,
- Examples: Smart homes, wearables in healthcare, industrial IoT (IIoT), smart cities, and connected vehicles.
Mobile Cloud Computing (MCC)[edit]
Mobile Cloud Computing (MCC) combines cloud computing and mobile phones to maximize processing power and storage. The system enables computation-intensive processing to be offloaded from mobile phones to cloud servers, thereby conserving battery life while improving performance.
Benefits of MCC:[edit]
- Extended Battery Life: Processing tasks are offloaded to the cloud, which reduces local energy consumption,
- Higher Processing Power: Allows sophisticated applications like AI and machine learning,
- Scalability: Dynamically adjusts with changing user loads,
- Real-Time Access: Provides access to cloud-hosted applications from remote locations
- Applications: Cloud-hosted AI personal assistants (like Google Assistant), cloud gaming, and AR/VR applications
Digital Twins[edit]
What Is a Digital Twin?[edit]
A Digital Twin is a virtual replica of a physical object, system, or process that is continuously updated with real-time data. It uses sensors, connectivity, and analytics to mirror and simulate the behavior and performance of its physical counterpart. This enables organizations to monitor, diagnose, predict, and optimize operations virtually before applying changes in the real world.
How Digital Twins Relate to IoT[edit]
The Internet of Things (IoT) plays a foundational role in enabling Digital Twin technology. IoT devices—such as sensors, actuators, smart meters, and wearables—collect data from the physical environment and stream it into the digital twin model in real time. Here’s how the connection works:
- Data Collection: IoT sensors gather metrics such as temperature, vibration, pressure, humidity, or motion,
- Data Transmission: These metrics are transmitted over a network to a central system,
- Real-Time Sync: The digital twin receives this data to update its model, reflecting the real-time status of the asset,
- Insight Generation: Analytics, AI/ML models, and simulations applied to the digital twin enable predictive maintenance, performance tuning, and scenario testing without affecting the physical system.
Practical Use Cases[edit]
- Smart Manufacturing: IoT-enabled machinery is mirrored by digital twins to optimize workflows, reduce downtime, and simulate design changes,
- Smart Cities: Digital twins of buildings, transport systems, or power grids leverage data from IoT sensors to improve efficiency and public safety,
- Healthcare: Wearables and connected medical devices provide biometric data to patient-specific digital twins for personalized treatment simulations.
Benefits:[edit]
- Real-Time Monitoring,
- Predictive Analytics & Maintenance
- Virtual Experimentation
- Enhanced Decision-Making
- Enhanced Decision-Making
This foundation makes it easy to later explore where digital twins should be deployed (edge vs cloud), based on latency, bandwidth, data sensitivity, and computational demand.
Digital twins are virtual representations of physical objects that are regularly updated with real-time data so as to replicate, predict, and maximize functionality. Digital twins are increasingly applied in manufacturing, healthcare, and smart city projects that enable intelligent cities, where predictive maintenance and systems optimization can be realized.
Digital twins connect physical devices with virtual spaces. Digital twins are run at edge nodes in edge computing to carry out local processing of information to minimize the necessity to send colossal amounts of data to the cloud. The configuration is especially applicable in cases requiring real-time analytics, for example, industrial automation and healthcare monitoring.
Diagram 1: Digital Twin Edge Network Architecture
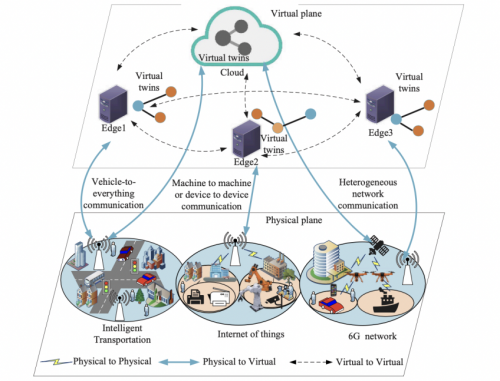
This diagram illustrates a layered architecture where virtual twins communicate with physical IoT devices through edge nodes. It showcases intelligent transportation systems, 6G networks, and IoT applications as part of a hierarchical edge-cloud framework.
2.2 Cloud[edit]
Cloud computing is an on-demand model of ubiquitous, easy, network access to shared pools of programmable computer infrastructure such as networks, servers, storage, software applications, and services that may be rapidly de-provisioned and provisioned with little administrative effort. Cloud computing provides access to high-bandwidth computational power without the necessity of owning and maintaining physical infrastructures.
Why the Continuum Will Dominate the Future[edit]
1. Proximity and Real-Time Responsiveness Edge computing brings computation closer to data sources—ideal for latency-sensitive applications such as autonomous vehicles, augmented reality, and real-time video analytics. The continuum optimizes where computation occurs—at the edge, near edge, or cloud—based on performance needs.
2. Scalability Across Diverse Infrastructures From smart homes to industrial automation to smart cities, edge devices vary widely in capabilities. The continuum provides a unified model to scale applications from small edge sensors to large cloud-hosted AI models.
3. Flexibility and Adaptability Workloads can dynamically shift based on resource availability, cost, network conditions, or privacy constraints. For instance, video streams can be pre-processed at the edge and fully analyzed in the cloud only when needed, reducing bandwidth usage and improving efficiency.
4. Support for Advanced Use Cases Emerging applications such as connected autonomous systems, Industry 4.0, and real-time federated learning rely on the interplay between edge and cloud. The continuum supports these by allowing AI, analytics, and orchestration tools to operate fluidly across environments.
5. Improved Security, Privacy, and Data Sovereignty The continuum allows sensitive data to be processed locally, enforcing data residency laws and reducing exposure to breaches. Meanwhile, global-scale analytics can be performed on anonymized or aggregated data in the cloud.
6. Optimized Resource Utilization and Cost Efficiency The continuum benefits from statistical multiplexing, allowing workloads to be scheduled and shifted to underutilized resources whether at the edge or in the cloud, enhancing overall utilization and reducing operational costs.
Technical Innovations:[edit]
- Federation Standards (e.g., IEEE 2302-2021): Enables trust and interoperability across diverse environments,
- Zero-Trust Security Models: Secure workloads dynamically across distributed nodes,
- Microservices and Containers: Allow modular, portable deployments across cloud and edge,
- Orchestration Platforms (like Kubernetes and KubeEdge): Manage application components across the continuum.
Broader Impact and Vision[edit]
The edge–cloud continuum supports global digital transformation. It’s essential to sectors like smart healthcare, national disaster response systems, autonomous transportation, and cyber-physical systems, all of which need reliability, responsiveness, and scalability that neither cloud nor edge can provide alone.
Characteristics of Cloud Computing:[edit]
- On-Demand Self-Service: Consumers may access computing capabilities on their own without human interference from the provider,
- Broad Network Access: The cloud services may be accessed from the network and are capable of supporting heterogeneous client platforms like laptop computers, cell phones, and workstations,
- Resource Pooling: The computer resources of the provider are collected to provide service to multiple consumers through a multi-tenant model,
- Rapid Elasticity: Abilities are provisioned elastically and also de-provisioned to grow quickly in response to demand,
- Measured Service: Resources utilization is metered, controlled, and reported, and offers visibility for the provider as well as the consumer.
Cloud Service Models:[edit]
- Infrastructure as a Service (IaaS): Offers raw computing resources like virtual machines, storage, and networks. AWS EC2 and Google Compute Engine are examples,
- Platform as a Service (PaaS): Platform as a Service (PaaS): Offers environments in which applications can be created, tested, and run. It covers up the underlying infrastructure, focusing on application development (e.g., AWS Elastic Beanstalk, Heroku)
- Software as a Service (SaaS): Delivers applications over the internet on a pay-per-use basis, without the need to install or maintain software (e.g., Microsoft 365, Salesforce).
Deployment Models:[edit]
- Public Cloud: Third-party suppliers manage it, offering services over the public internet. For low-security needs and high scalability,
- Private Cloud: Dedicated for use by one organization, offering more control and security. Installed on-premises or by a third party,
- Hybrid Cloud: Combines public and private clouds, enabling sharing of applications and data between them,
- Multi-Cloud: Utilizes services from multiple cloud vendors to avoid vendor lock-in and enhance reliability.
Challenges in Cloud Computing:[edit]
- Latency Problems: Cloud processing may lead to delays, especially in real-time systems,
- Privacy of Data: Transmission of data to the cloud can be a security risk,
- Cost of Bandwidth: Ongoing data transfer between equipment and the cloud is costly,
- Outages and Downtime: Interruptions in cloud services affect availability.
Integration of Cloud and Edge:[edit]
Edge computing is superior to cloud computing because it computes closer to the source and leverages the cloud for intense analytics and data storage. An example would be when in autonomous vehicles, local real-time calculation (i.e., object detection) happens while machine learning model training data are sent to the cloud.
Diagram 2: Edge Computing Architecture
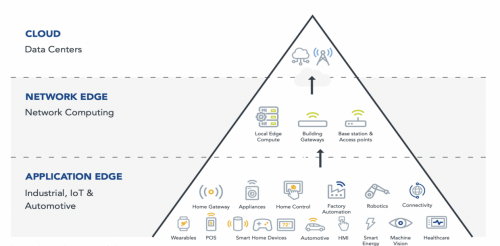
This diagram represents the layered structure of edge computing, highlighting how the cloud layer interacts with network and application edges. The cloud primarily handles heavy computation and data storage, while edge devices focus on local data processing.
2.3 Edge and Fog[edit]
Edge Computing:[edit]
Edge computing handles data at or close to the place where the data is situated, minimizing the latency in transmitting data to the cloud servers centrally. It is critical for applications that need real-time data processing and response, including autonomous vehicles, industrial automation, and medical monitoring.
- Reduced Latency: Edge computing reduces the latency of transmitting data over long distances as it processes the data locally.
- Improved Data Privacy: Personal data is processed closer to the source, reducing the risk of exposure.
- Bandwidth Efficiency: There is only transmission of processed data or key information to the cloud, which minimizes data traffic,
- High Reliability: Edge devices can operate independently even when the network connectivity with the cloud is severed.
Applications of Edge Computing:[edit]
- 'Autonomous Vehicles: Real-time data processing for navigation and obstacle avoidance,
- Smart Cities: Adaptive lighting networks and traffic monitoring,
- Healthcare: Real-time remote monitoring equipment to analyze patients' information,
- Industrial IoT: Predictive maintenance through sensor data.
Fog Computing:[edit]
Fog computing is an extension of edge computing in the way that it creates a middle layer between the cloud and the edge devices. Fog computing enables data to be collected, computed, and decision-making performed nearer to the data source but not on the device itself.
Features of Fog Computing:[edit]
- Intermediate Processing: Relieves edge devices of some processing tasks prior to reaching the cloud,
- Data Aggregation: It combines data from various sources to eliminate redundancy,
- Enhanced Scalability: Enables massive IoT networks by distributing computation tasks
- Real-Time Analytics: Processes real-time data in real time without depending on distant servers
Comparison: Edge vs. Fog:[edit]
- Processing Location: Edge directly on devices; Fog on intermediate nodes
- Latency: Edge has ultra-low latency; Fog has low but comparatively higher latency
- Scalability: Fog is more scalable because of the capability of data combining
- Data Aggregation: Edge deals with individual data, while Fog consolidates data from multiple sources.
Diagram 3: Edge-Fog-Cloud Network Architecture
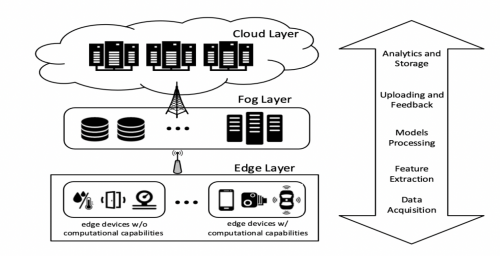
The diagram shows the interplay between cloud, fog, and edge layers, emphasizing the data flow from localized edge processing through fog nodes to the centralized cloud for deeper analytics.
2.4 Edge-Cloud Continuum[edit]
Edge-Cloud Continuum is defined as an adaptive and dynamic computational paradigm that balances edge, fog, and cloud computation workloads. Through this integration of environments, applications can toggle between local and remote processing depending on workload, latency constraints, and network status.
Core Functions:[edit]
- Dynamic Workload Distribution: Real-time tasks are processed at the edge, while less critical operations are pushed to the cloud,
- Real-Time Data Processing: Edge devices manage time-sensitive data, like sensor readings, without sending it to remote servers
- Data Filtering and Aggregation: Fog nodes filter data before forwarding it to the cloud, minimizing data transfer
- Scalable and Elastic Resource Management: Allocates resources adaptively according to the computational demand
- Security and Privacy Management: Implements localized data encryption and access control,
- Interoperability: Facilitates smooth interaction between edge, fog, and cloud systems through intelligent orchestration.
Applications:[edit]
- Smart Grids: Real-time monitoring and load balancing between local power sources and centralized control
- Smart Agriculture: Local analysis of environmental data, with broader data analytics performed in the cloud
- Healthcare Monitoring: Real-time patient monitoring at the edge, with historical analysis in the cloud
By blending edge and cloud processing, the continuum provides a flexible infrastructure that adapts to real-time requirements while maintaining efficient long-term data management.