Federated Learning
Overview and Motivation
Federated Learning (FL) is a decentralized machine learning paradigm that enables multiple edge devices referred to as clients to collaboratively train a shared model without transferring their private data to a central location. Each client performs local training using its own dataset and communicates only model updates (such as gradients or weights) to an orchestrating server or aggregator. These updates are then aggregated to produce a new global model that is redistributed to the clients for further training. This process continues iteratively, allowing the model to learn from distributed data sources while preserving the privacy and autonomy of each client. By design, FL shifts the focus from centralized data collection to collaborative model development, introducing a new direction in scalable, privacy-preserving machine learning [1].
The motivation for Federated Learning arises from growing concerns around data privacy, security, and communication efficiency particularly in edge computing environments where data is generated in massive volumes across geographically distributed and often resource-constrained devices. Centralized learning architectures struggle in such contexts due to limited bandwidth, high transmission costs, and strict regulatory frameworks such as the General Data Protection Regulation (GDPR) and the Health Insurance Portability and Accountability Act (HIPAA). FL inherently mitigates these issues by allowing data to remain on-device, thereby minimizing the risk of data exposure and reducing reliance on constant connectivity to cloud services. Furthermore, by exchanging only lightweight model updates instead of full datasets, FL significantly decreases communication overhead, making it well-suited for real-time learning in mobile and edge networks [2].
Within the broader ecosystem of edge computing, FL represents a paradigm shift that enables distributed intelligence under conditions of partial availability, device heterogeneity, and non-identically distributed (non-IID) data. Clients in FL systems can participate asynchronously, tolerate network interruptions, and adapt their computational loads based on local capabilities. This flexibility is particularly important in edge scenarios where devices may differ in processor power, battery life, and storage. Moreover, FL supports the development of personalized and locally adapted models through techniques such as federated personalization and clustered aggregation. These properties make FL not only an effective solution for collaborative learning at the edge but also a foundational approach for building scalable, secure, and trustworthy AI systems that are aligned with emerging demands in distributed computing and privacy-preserving technologies [1][2][3].
Federated Learning Architectures
Federated Learning (FL) can be implemented through various architectural configurations, each defining how clients interact, how updates are aggregated, and how trust and responsibility are distributed. These architectures play a central role in determining the scalability, fault tolerance, communication overhead, and privacy guarantees of a federated system. In edge computing environments, where client devices are heterogeneous and network reliability varies, the choice of architecture significantly affects the efficiency and robustness of learning. The three dominant paradigms are centralized, decentralized, and hierarchical architectures. Each of these approaches balances different trade-offs in terms of coordination complexity, system resilience, and resource allocation.
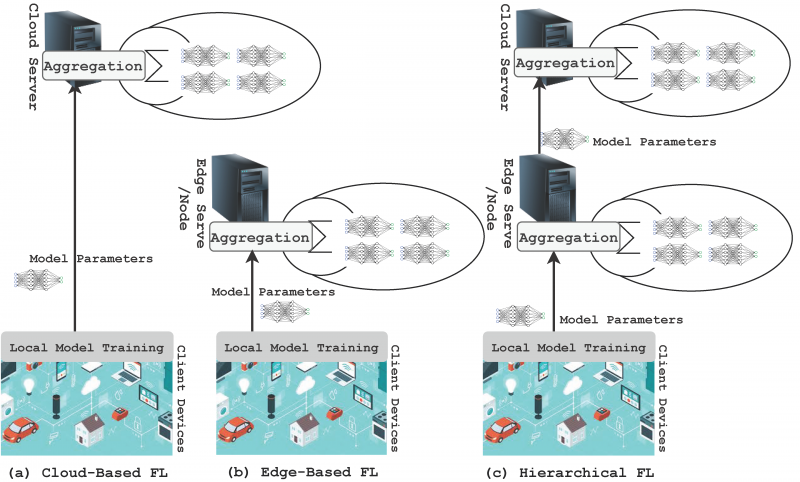
Centralized Architecture
In the centralized FL architecture, a central server or cloud orchestrator is responsible for all coordination, aggregation, and distribution activities. The server begins each round by broadcasting a global model to a selected subset of client devices, which then perform local training using their private data. After completing local updates, clients send their modified model parameters usually in the form of weight vectors or gradients back to the server. The server performs aggregation, typically using algorithms such as Federated Averaging (FedAvg), and sends the updated global model to the clients for the next round of training.
The centralized model is appealing for its simplicity and compatibility with existing cloud to client infrastructures. It is relatively easy to deploy, manage, and scale in environments with stable connectivity and limited client churn. However, its reliance on a single server introduces critical vulnerabilities. The server becomes a bottleneck under high communication loads and a single point of failure if it experiences downtime or compromise. Furthermore, this architecture requires clients to trust the central aggregator with metadata, model parameters, and access scheduling. In privacy-sensitive or high availability contexts, these limitations can restrict centralized FL’s applicability [1].
Decentralized Architecture
Decentralized FL removes the need for a central server altogether. Instead, client devices interact directly with each other to share and aggregate model updates. These peer-to-peer (P2P) networks may operate using structured overlays, such as ring topologies or blockchain systems, or employ gossip-based protocols for stochastic update dissemination. In some implementations, clients collaboratively compute weighted averages or perform federated consensus to update the global model in a distributed fashion.
This architecture significantly enhances system robustness, resilience, and trust decentralization. There is no single point of failure, and the absence of a central coordinator eliminates risks of aggregator bias or compromise. Moreover, decentralized FL supports federated learning in contexts where participants belong to different organizations or jurisdictions and cannot rely on a neutral third party. However, these benefits come at the cost of increased communication overhead, complex synchronization requirements, and difficulties in managing convergence, especially under non-identical data distributions and asynchronous updates. Protocols for secure communication, update verification, and identity authentication are necessary to prevent malicious behavior and ensure model integrity. Due to these complexities, decentralized FL is an active area of research and is best suited for scenarios requiring strong autonomy and fault tolerance [2].
Hierarchical Architecture
Hierarchical FL is a hybrid architecture that introduces one or more intermediary layers—often called edge servers or aggregators between clients and the global coordinator. In this model, clients are organized into logical or geographical groups, with each group connected to an edge server. Clients send their local model updates to their respective edge aggregator, which performs preliminary aggregation. The edge servers then send their aggregated results to the cloud server, where final aggregation occurs to produce the updated global model.
This multi-tiered architecture is designed to address the scalability and efficiency challenges inherent in centralized systems while avoiding the coordination overhead of full decentralization. Hierarchical FL is especially well-suited for edge computing environments where data, clients, and compute resources are distributed across structured clusters, such as hospitals within a healthcare network or base stations in a telecommunications infrastructure.
One of the key advantages of hierarchical FL is communication optimization. By aggregating locally at edge nodes, the amount of data transmitted over wide-area networks is significantly reduced. Additionally, this model supports region-specific model personalization by allowing edge servers to maintain specialized sub-models adapted to local client behavior. Hierarchical FL also enables asynchronous and fault-tolerant training by isolating disruptions within specific clusters. However, this architecture still depends on reliable edge aggregators and introduces new challenges in cross-layer consistency, scheduling, and privacy preservation across multiple tiers [1][3].
Aggregation Algorithms and Communication Efficiency
Aggregation is a fundamental operation in Federated Learning (FL), where updates from multiple edge clients are merged to form a new global model. The quality, stability, and efficiency of the federated learning process depend heavily on the aggregation strategy employed. In edge environments characterized by device heterogeneity and non-identical data distributions choosing the right aggregation algorithm is essential to ensure reliable convergence and effective collaboration.
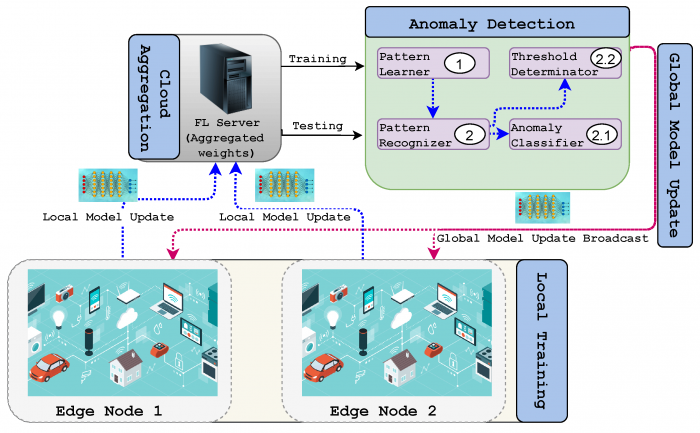
Key Aggregation Algorithms
Algorithm | Description | Handles Non-IID Data | Server-Side Optimization | Typical Use Case |
---|---|---|---|---|
FedAvg | Performs weighted averaging of client models based on dataset size. Simple and communication-efficient. | Limited | No | Basic federated setups with IID or mildly non-IID data. |
FedProx | Adds a proximal term to the local loss function to prevent client drift. Stabilizes training with diverse data. | Yes | No | Suitable for edge deployments with high data heterogeneity or resource-limited clients. |
FedOpt | Applies adaptive optimizers (e.g., FedAdam, FedYogi) on aggregated updates. Enhances convergence in dynamic systems. | Yes | Yes | Used in large-scale systems or settings with unstable participation and gradient variability. |
Aggregation is the cornerstone of Federated Learning (FL), where locally computed model updates from edge devices are combined into a global model. The most widely adopted aggregation method is Federated Averaging (FedAvg), introduced in the foundational work by McMahan et al. FedAvg operates by averaging model parameters received from participating clients, typically weighted by the size of each client’s local dataset. This simple yet powerful method reduces the frequency of communication by allowing each device to perform multiple local updates before sending gradients to the server. However, FedAvg performs optimally only when data across clients is balanced and independent and identically distributed (IID)—conditions rarely satisfied in edge computing environments, where client datasets are often highly non-IID, sparse, or skewed [1][2].
To address these limitations, several advanced aggregation algorithms have been proposed. One notable extension is FedProx, which modifies the local optimization objective by adding a proximal term that penalizes large deviations from the global model. This constrains local training and improves stability in heterogeneous data scenarios. FedProx also allows flexible participation by clients with limited resources or intermittent connectivity, making it more robust in practical edge deployments. Another family of aggregation algorithms is FedOpt, which includes adaptive server-side optimization techniques such as FedAdam and FedYogi. These algorithms build on optimization methods used in centralized training and apply them at the aggregation level, enabling faster convergence and improved generalization under complex, real-world data distributions. Collectively, these variants of aggregation address critical FL challenges such as slow convergence, client drift, and update divergence due to heterogeneity in both data and device capabilities [1][3].
Communication Efficiency in Edge-Based FL
Communication remains one of the most critical bottlenecks in deploying FL at the edge, where devices often suffer from limited bandwidth, intermittent connectivity, and energy constraints. To address this, several strategies have been developed. **Gradient quantization** reduces the size of transmitted updates by lowering numerical precision (e.g., from 32-bit to 8-bit values). **Gradient sparsification** limits communication to only the most significant changes in the model, transmitting top-k updates while discarding negligible ones. **Local update batching** allows devices to perform multiple rounds of local training before sending updates, reducing the frequency of synchronization.
Further, **client selection strategies** dynamically choose a subset of devices to participate in each round, based on criteria like availability, data quality, hardware capacity, or trust level. These communication optimizations are crucial for ensuring that FL remains scalable, efficient, and deployable across millions of edge nodes without overloading the network or draining device batteries [1][2][3].
Privacy Mechanisms
Federated Learning (FL) was introduced to decentralize model training while preserving privacy by design. However, privacy risks still exist because shared model updates can leak information about local data. This is particularly important in edge-based applications, such as smart healthcare or autonomous vehicles, where sensitive and personal data resides on resource-constrained IoT devices. To counter this, a variety of privacy-preserving mechanisms have been developed to enhance FL’s trustworthiness while maintaining utility. These mechanisms fall into categories such as differential privacy, secure aggregation, homomorphic encryption, and secure multi-party computation, each offering unique trade-offs.
Differential Privacy (DP)
Differential Privacy introduces random noise into model updates before they are sent from the client to the server. The goal is to ensure that the presence or absence of a single user’s data does not significantly affect the output of the learning algorithm. In practice, this is often implemented by adding Gaussian or Laplace noise to gradients or parameters. While DP guarantees strong mathematical privacy, the addition of noise can degrade model accuracy, especially when privacy budgets are tight. To maintain balance, adaptive privacy budgeting and gradient clipping are often applied. In edge scenarios like personalized health data processing on smartphones, DP can help ensure regulatory compliance with privacy laws such as GDPR and HIPAA [1][2].
Secure Aggregation
Secure Aggregation (SecAgg) is designed to prevent the central server from accessing individual client updates by ensuring that only the aggregate of all updates can be decrypted. This is achieved through cryptographic techniques where clients mask their updates using pairwise keys or secret shares, which cancel out during aggregation. The result is that the server learns only the sum of the updates but not any single client’s data. This is particularly effective when used in environments with many participating IoT devices, such as vehicle nodes in autonomous driving networks or sensors in smart cities. Secure aggregation is compatible with FL algorithms like FedAvg and is often paired with other techniques like client selection or update thresholding to maintain efficiency [2][3].
Homomorphic Encryption (HE)
Homomorphic Encryption allows mathematical operations to be performed directly on encrypted data without decrypting it. In FL, this means that clients can encrypt their model updates before transmission, and the server can compute an aggregated model without ever seeing the raw updates. Although conceptually appealing, HE is computationally expensive and typically limited to simple arithmetic operations, making it challenging for deep learning models on edge devices. Nonetheless, it can be used effectively in cross-silo FL where clients are institutions (e.g., hospitals) with greater computational power. Use cases like federated diagnostics across multiple hospitals benefit from HE without compromising data ownership or privacy [1][3].
Secure Multi-Party Computation (SMPC)
Secure Multi-Party Computation enables multiple clients to jointly compute a function over their inputs while keeping those inputs private. In FL, SMPC can be used to distribute trust across clients so that no single entity holds the entire view of the training data or model updates. This decentralization is especially useful in peer-to-peer or blockchain-backed federated learning setups. While SMPC provides strong privacy guarantees, it introduces communication overhead and synchronization complexity, making it more suitable for federations of powerful edge servers rather than lightweight IoT clients [1][2].
Integration in Edge Environments
In edge computing, privacy mechanisms must be resource-efficient and resilient to unreliable network conditions. Techniques like local differential privacy are useful when clients (e.g., mobile phones or home routers) are intermittently connected or offline. Moreover, hierarchical edge architectures enable partial aggregation and privacy enforcement at intermediary nodes such as edge gateways. Privacy-preserving FL is essential in applications like smart transportation systems where location data, driving habits, and real-time decisions are sensitive and must remain private while still contributing to a shared intelligence network [2][3].