Emerging Research Directions: Difference between revisions
Line 124: | Line 124: | ||
|- | |- | ||
| KITE | | KITE | ||
| Quickly | | Quickly Assembled from Existing Hardware, Initialization is Simple | ||
| Algorithm for | | Algorithm for Mesh Reconstruction is not accurate and Stable | ||
|- | |- | ||
| Nexus | | Nexus | ||
| Spatial-aware | | Spatial-aware Applications, Easy and Common Infrastructure | ||
| Needs to | | Needs to Stores and Manages the Location Information Globally | ||
|- | |- | ||
| Vuforia | | Vuforia | ||
| Most widely | | Most widely Used, Tracking is Flicker-less | ||
| Paid | | Paid License is Required for advance AR Features | ||
|- | |- | ||
| WARP | | WARP | ||
| A well defined Layered Structure architecture | | A well defined Layered Structure architecture | ||
| Relatively | | Relatively Slower Processing | ||
|- | |- | ||
| Wikitude | | Wikitude | ||
| Geo-based | | Geo-based Tracking Available | ||
| License | | License Needed for the App Development | ||
|} | |} | ||
Revision as of 22:58, 19 April 2025
Emerging Research Directions
7.1 Task and Resource Scheduling
7.1.1 Introduction
Task and resource scheduling in edge computing aims to tackle issues of latency reduction, energy efficiency, and resource optimization by intelligently coordinating the architecture and configuration of the edge computing system. According to [1], the factors can be summarized into the following list:
- Resources: hardware and software capabilities that provide communication, storage/caching, and computing
- Tasks: high-level applications of the systems, such as safety, health monitoring, security, etc.
- Participants: the computational components that can collaborate with one another, i.e., users/”things”, edge, and cloud
- Objectives: low-level applications of the systems, e.g., lower latency for traffic safety, reduce energy consumption for device longevity, etc.
- Actions: methods to achieve the objectives, e.g., computation offloading, resource allocation, resource provisioning
- Methodology: strategies to best tackle the actions mentioned above, categorized as centralized and decentralized
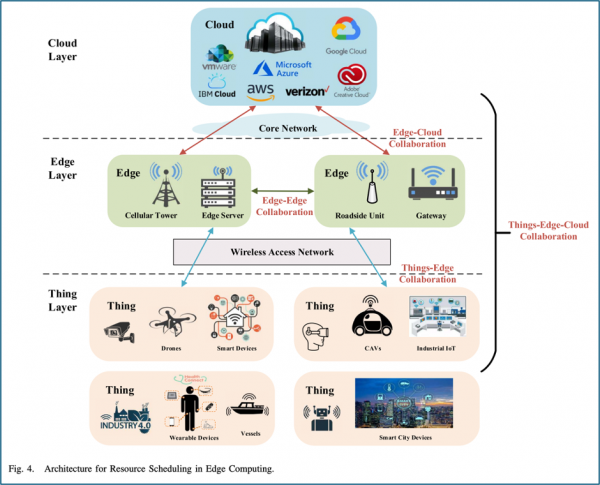
7.1.2 Core Challenges in Scheduling
The main challenges in task and resource scheduling are primarily the heterogeneous and constrained edge resources that these types of systems work with, the dynamic workloads and real-time requirements that they are deployed in, and privacy and security concerns. On the first point, not only are the devices heterogenous and geographically distributed, the data volume and data attributes are also heterogenous. While challenging, resource/task scheduling can reduce the difficult effects of this aspect of edge computing.
In terms of the dynamic workloads and real-time requirements, the main complication stems from user mobility with their devices. Smartphones, connected vehicles (CVs), and other mobile devices are often moving through the world connecting to different nodes over various protocols. Per [2], strategies that determine offloading and cache decisions can be incorrect very quickly after deciding, or its users may move out of range, or into range of a separate setup.
For security and privacy, the main challenges are categorized as such: system-level, service-level, and data-level. System-level discusses the overall reliability of the edge system, whether by intrusion or malfunction. Service-level discusses user authentication/authorization and validation of offload nodes. Lastly, data-level discusses the trustworthiness and protection of the data as it passes between IoT devices and edge nodes, as the data can contain sensitive information.
7.1.3 Scheduling Strategies and Algorithms
The task/resource scheduling can be split between centralized methods vs. distributed methods. Examples of centralized methods include convex optimization, approximate algorithm, heuristic algorithm, and machine learning. Examples of distributed methods include game theory, matching theory, auction, federated learning, and blockchain. The effectivity of each method can be measured by the following performance indicators: latency, energy consumption, cost, utility, profit, and resource utilization [1].
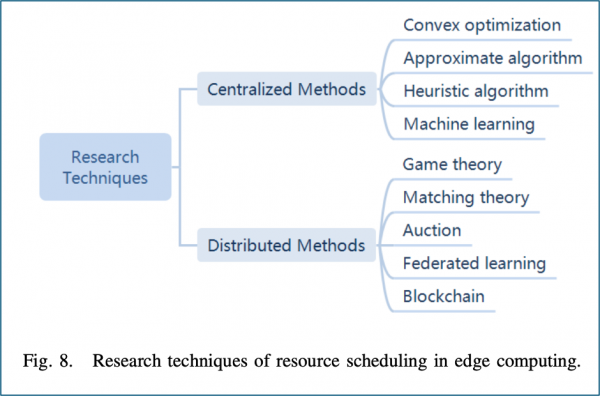
7.1.4 Edge-Cloud Collaboration
- Task offloading strategies and decision models
- Computational perspective
- Centralized, decentralized, distributed
- Decision-making perspective
- Cloud-assisted, ue driven, sdn based, collaborative
- Algorithmic paradigm
- Greedy heuristics, integer programming, machine learning, branch and bound, dynamic programming, convex optimization
- Computational perspective
- Hybrid scheduling frameworks for improved performance
7.1.5 Future Trends and Research Directions
- Federated learning for decentralized scheduling
- Green computing and sustainability considerations
- “Although marvelous solutions are proposed in existing works, most of them consider the extra energy can be harvested continuously [72], [186]. However, in practice, the energy harvesting process may be unstable, which poses a significant challenge in designing an efficient resource scheduling strategy.”
- Open challenges in adaptive and autonomous scheduling
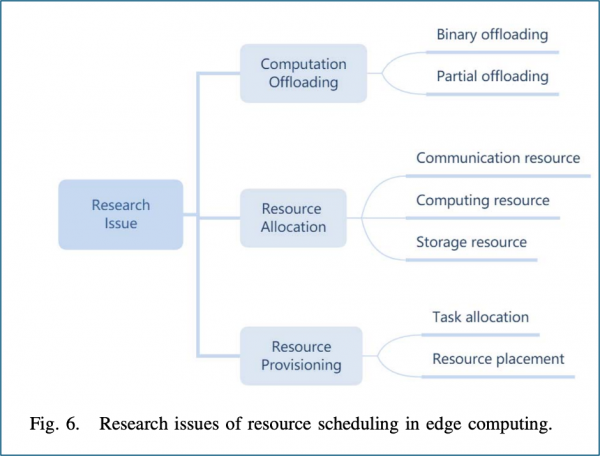
7.1.6 Conclusion
- Key takeaways and impact of scheduling on edge ecosystems
References
[1] Luo, Quyuan, et al. "Resource scheduling in edge computing: A survey." IEEE communications surveys & tutorials 23.4 (2021): 2131-2165.
[2] Lin, Li, et al. "Computation offloading toward edge computing." Proceedings of the IEEE 107.8 (2019): 1584-1607.
[3] Jiang, Congfeng, et al. "Energy aware edge computing: A survey." Computer Communications 151 (2020): 556-580.
[4] Khan, Wazir Zada, et al. "Edge computing: A survey." Future Generation Computer Systems 97 (2019): 219-235.
[5] Islam, Akhirul, et al. "A survey on task offloading in multi-access edge computing." Journal of Systems Architecture 118 (2021): 102225.
7.2 Edge for AR/VR
Introduction
As Edge Computing expands into new opportunities and utilizations in our society, so too does it reach Augmented Reality (AR) and Virtual Reality (VR) devices. From simple visualization needs to advancements in surgery, society has only begun to grasp the possibilities development of such technology may hold.
AR, What Is It?
Augmented Reality (AR) is a technology that enhances a user’s real-world experience through digital features.
The Four AR Layers
Types of AR
AR Frameworks and Platforms
Platform | Positive Features | Negative Features |
---|---|---|
ARBlocks | Good Visualization and Tracking | Relatively Slow |
ARCore | Good Understanding of the Environment, Good for Gaming | AR Tracking is not consistent, Not Stable on Older Generation Devices |
ARKit | Good AR Rendering Devices | Charges for App Distribution |
AR-media | Offers Cloud Based Services for AR | Depth mapping is not Available Yet |
ARToolKit | Processing happens in real time | Can be used only with image markers. |
ARWin | Interact in the Virtual Environment | Careful Calibration of Markers is Required |
Bright | Comfortable AR HMDs | Slow Processing as it is Done Locally |
CoVAR | Eye-Gaze, Head-Gaze, and Hand Gestures Based Interaction | Simulator Sickness |
DUIRA | Create a Realistic Virtual Digital Environment that Reflects Real-World Experiences | Requires Multiple AR Markers |
KITE | Quickly Assembled from Existing Hardware, Initialization is Simple | Algorithm for Mesh Reconstruction is not accurate and Stable |
Nexus | Spatial-aware Applications, Easy and Common Infrastructure | Needs to Stores and Manages the Location Information Globally |
Vuforia | Most widely Used, Tracking is Flicker-less | Paid License is Required for advance AR Features |
WARP | A well defined Layered Structure architecture | Relatively Slower Processing |
Wikitude | Geo-based Tracking Available | License Needed for the App Development |
Challenges Faced by AR
Edge for AR
VR, What Is It?
Features of VR
Types of VR
Challenges Faced by VR
Edge for VR
References
7.3 Vehicle Computing
Introduction
The rapid advancement in intelligent transportation systems (ITS), autonomous driving, and vehicle-to-everything (V2X) communication has made edge computing an indispensable enabler of innovation. As vehicles become increasingly autonomous, the need for fast, reliable, and low-latency processing grows. Edge computing, by decentralizing data processing and moving it closer to the data source, is revolutionizing how vehicles interact with their environment. This chapter explores the growing field of vehicle edge computing (VEC), analyzing its current role, challenges, and emerging use cases. We synthesize insights from industry presentations, academic research, and real-world implementations to provide a cohesive narrative of the transformative impact of edge computing on the transportation ecosystem.
Overview of Edge Computing
Edge computing refers to the deployment of computing services closer to data sources such as sensors, actuators, or connected devices. Unlike traditional cloud computing, which relies on remote data centers, edge computing reduces latency, saves bandwidth, and enhances real-time decision-making capabilities.
Key Benefits for Automotive Applications:
- Ultra-low latency: Enables real-time decision-making for safety-critical functions.
- Bandwidth optimization: Reduces data transmission by processing information locally.
- Improved privacy and security: Minimizes data exposure by localizing computation.
- Increased reliability: Maintains operation in areas with intermittent connectivity.
2. The Need for Edge in Vehicles
2.1. Data Explosion in Connected Vehicles Modern vehicles are equipped with over 300 sensors and generate terabytes of data daily. As noted by Shi et al. [1], a connected vehicle can produce up to 35 TB of data per day. Centralized cloud architectures are insufficient to handle such high-throughput data streams efficiently.
2.2. Latency and Safety Latency is critical in autonomous systems. In traditional architectures, a vehicle may need to send sensor data to the cloud, await processing, and receive instructions—a delay that could be fatal in scenarios like pedestrian detection. "By the time the information has traveled to and from the server, the accident has already occurred." Edge computing enables immediate action, reducing the delay to milliseconds.
2.3. Software-Defined Vehicles The vehicle industry is moving towards software-defined vehicles (SDVs), which require OTA (Over-The-Air) updates, real-time diagnostics, and modular software services. Edge computing facilitates dynamic service delivery, AI inference, and adaptive updates without overwhelming the cloud infrastructure.
3. Architectures for Vehicle Edge Computing
3.1. Multi-Tiered Architecture Vehicle edge computing generally involves a four-layer architecture:
- On-board Vehicle Computing (VEC): Direct AI inference and sensor processing.
- Edge Servers (e.g., RSUs, roadside units): Shared compute resources at intersections or base stations.
- IoT Layer: Includes environmental sensors and auxiliary connected devices.
- Cloud Tier: For large-scale analytics, simulations, and HD map updates.
3.2. Real-World Example: Autonomous Vehicle Stack
Autonomous driving systems like those developed by Waymo and Uber use a distributed computing model:
- Local x86 nodes with GPUs and FPGAs on the vehicle.
- Sensors (LIDAR, radar, cameras) feed into real-time AI models.
- Local control software performs path planning and object avoidance.
Figure 1: [Vehicle Stack from Uber AV System – Custom Compute Nodes, Sensor Interfaces, Telematics Modules. Source: Seminar Presentation.]
4. Emerging Use Cases in Vehicle Edge Computing
4.1. Autonomous Driving Autonomous vehicles (AVs) rely on edge computing to interpret sensor data in real-time. They use:
- LIDAR and radar for object detection.
- Local AI models for path planning.
- Embedded GPUs/ASICs for deep learning inference.
Edge computing enables AVs to operate safely without full cloud reliance. Simulation systems allow for massive scenario-based testing, further validating algorithms [Seminar Presentation].
4.2. Predictive Maintenance In the user's final project [2], edge computing was leveraged for preventive maintenance warnings using AI models deployed on vehicle microcontrollers. These systems: Monitor signals from OBD-II interfaces. Detect early anomalies in vibration, RPM, or temperature. Alert drivers before mechanical failures occur. This showcases how edge AI can extend vehicle life and improve safety.
4.3. Smart Infrastructure Monitoring Connected vehicles can serve as mobile sensors for cities. Shi [1] highlights how they: Detect potholes or damaged infrastructure. Share data with city edge servers for repair scheduling. Enable crowdsourced infrastructure diagnostics. This creates a synergistic ecosystem between vehicles and smart cities.
4.4. Safety and Law Enforcement Edge-powered applications include: Real-time passenger behavior monitoring for ride-sharing. Situational awareness for police vehicles using AI and camera feeds. Edge-assisted bodycam processing for faster incident reporting.
4.5. Enhanced V2X Communication with Beamforming Using dynamic radar-assisted beamforming, vehicle-to-infrastructure (V2I) communications can adaptively focus beams toward edge units based on detected obstacles [3]. This ensures: Reliable data transmission under high mobility. Better handling of occlusions and multi-path scenarios. Figure 2: [Illustration of Beamforming System in V2I from Paper [3]]
5. Technical Challenges and Research Directions
5.1. Heterogeneity and Resource Constraints Vehicles use different CPUs, GPUs, and network modules. Developing uniform SDKs and runtime environments (like the VPI mentioned by Shi) is crucial.
5.2. Real-Time OS and Scheduling Due to safety requirements, OS-level guarantees are needed for tasks like braking, detection, or communication. Time-sensitive networking and real-time scheduling are active research areas.
5.3. Data Offloading and Bandwidth Optimization Not all data can be offloaded to the cloud. Techniques like compressed AI inference, model partitioning, and log prioritization are necessary to reduce bandwidth costs.
5.4. Security and Privacy With increased vehicle connectivity comes increased risk. Secure boot, sandboxing, trusted execution environments, and decentralized authentication are vital in VEC systems.
5.5. Standardization and APIs There is a need for standardized vehicle-to-cloud and vehicle-to-infrastructure APIs to enable third-party service integration.
6. Future Vision and Business Implications
6.1. Vehicles as Edge Platforms Just like smartphones, vehicles will host apps that: Provide ride-sharing capabilities. Monitor infrastructure. Run entertainment or diagnostic services. OEMs could open SDKs for 3rd-party app development, creating a new vehicle app economy.
6.2. Distributed AI at the Edge Future systems will involve collaborative intelligence, where vehicles communicate with other vehicles and infrastructure to jointly solve navigation, perception, and safety problems.
6.3. Greener Cities and Sustainable Transport Edge-driven predictive maintenance, optimized routing, and dynamic parking can reduce fuel usage and improve traffic flow, supporting sustainability goals. Figure 3: [Four-Tier Architecture of Vehicle Computing – Source: Shi et al. [1]]
Conclusion
Edge computing is set to redefine the automotive landscape. By enabling real-time, decentralized intelligence, it supports safer, smarter, and more efficient transportation. From predictive maintenance and autonomous navigation to infrastructure monitoring and V2X communication, edge computing is the foundation of tomorrow’s intelligent mobility ecosystem. As research and development continue, addressing challenges such as heterogeneity, security, and scalability will be essential. The vehicle edge computing paradigm not only presents technical innovation but also opens doors for new business models, societal benefits, and academic exploration.
References
- Weisong Shi et al., "Vehicle Computing: Vision and Challenges," 2023.
- Mohamed Aboulsaad, "Preventive Maintenance Warning in Vehicles by Using AI," Final Project, 2024.
- Dynamic Beamforming for Vehicle-to-Infrastructure MIMO Communication Assisted by Sensing: Extended Target Case.
7.4 Energy-Efficient Edge Architectures
The exponential growth of the Internet of Things (IoT) devices, coupled with the emergence of artificial intelligence (AI) and high-speed communication networks (5G/6G), has led to the proliferation of edge computing. In an edge computing paradigm, data processing is distributed away from centralized cloud data centers and relocated closer to the data source or end-users. This architectural shift offers benefits such as reduced network latency, efficient bandwidth usage, and real-time analytics. However, the distribution of processing to a multiplicity of geographically dispersed devices has profound implications for energy consumption. Although large-scale data centers have been the subject of extensive research concerning energy efficiency, smaller edge nodes—including micro data centers, IoT gateways, and embedded systems—also generate significant carbon emissions [1].
High-Level Edge-Fog-Cloud Architecture
Modern IoT and AI systems often rely on an edge-fog-cloud architecture. Data collected by IoT sensors typically undergoes initial processing at edge nodes or micro data centers. This local processing minimizes the data volume that must be sent to the cloud, thereby reducing network congestion and latency. Intermediate fog nodes can then aggregate data from multiple edge devices for further analysis or buffering, while centralized cloud data centers handle large-scale storage and intensive computational tasks [2].
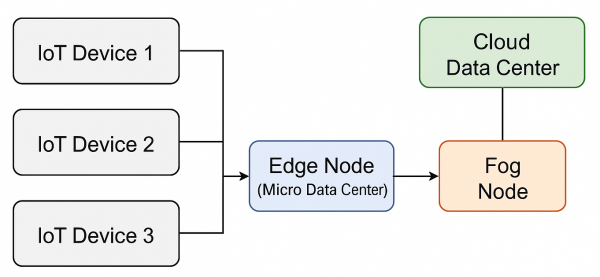
Lifecycle of an Edge Device
Evaluating the carbon footprint of edge devices requires considering their entire lifecycle. The manufacturing phase often involves substantial energy consumption and the use of raw materials. During deployment, the energy efficiency of operation including effective cooling, is critical. Ongoing maintenance and updates can extend a device's lifespan, while end-of-life disposal or recycling presents further environmental challenges. Each stage of the lifecycle offers opportunities for reducing carbon emissions through measures such as modular upgrades, the use of recycled materials, and environmentally responsible disposal practices [3].
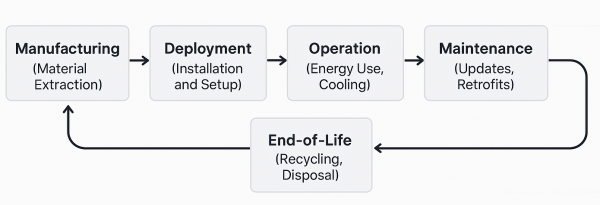
Hardware-Level Approaches
Research on hardware-focused strategies for reducing the carbon footprint at the edge has been extensive. Xu et al. examined system-on-chips (SoCs) designed specifically for energy efficiency, integrating ultra-low-power states and selective core activation [4]. Mendez and Ha evaluated heterogeneous multicore processors for embedded systems, highlighting the benefits of activating only the cores necessary to meet real-time performance requirements [5]. Similarly, the introduction of custom AI accelerators has been shown to yield significant power savings for neural network inference tasks [6].
Bae et al. emphasized that sustainable manufacturing practices and the use of recycled materials can reduce the overall lifecycle emissions of edge devices [7]. Kim et al. explored biologically inspired materials to enhance heat dissipation at the package level, while Liu and Zhang demonstrated that compact liquid-cooling solutions are viable even for micro data centers near the edge [8][9].
Study | Key Focus | Contributions | Findings |
---|---|---|---|
[4] Xu et al. | Ultra-low-power SoC design | Introduced SoC with power gating and selective core activation | Significant reduction in idle power consumption |
[5] Mendez and Ha | Heterogeneous multicore processors | Evaluated activating only necessary cores for real-time tasks | Improved balance of performance and energy usage |
[6] Ramesh et al. | Custom AI accelerators | Developed specialized hardware for on-device inference | Reported substantial energy savings in neural network operations |
[7] Bae et al. | Sustainable manufacturing | Employed lifecycle assessments and recycled materials | Achieved measurable decrease in manufacturing emissions |
[8] Kim et al. | Biologically inspired packaging | Integrated biomimetic materials for enhanced heat dissipation | Reduced cooling energy overhead and improved thermal performance |
[9] Liu and Zhang | Liquid cooling solutions | Demonstrated compact liquid-cooling viability in micro data centers | Quantitative improvement in cooling efficiency |
Software-Level Optimizations
Energy-aware software design is integral to achieving sustainability in edge computing. Wan et al. initiated the discussion on applying dynamic voltage and frequency scaling (DVFS) within edge-based real-time systems [10]. Martinez et al. refined DVFS strategies by incorporating reinforcement learning methods that adaptively tune voltage and frequency according to workload fluctuations, illustrating substantial improvements in power efficiency [11]. On the task scheduling front, Li et al. proposed multi-objective algorithms to distribute computing workloads among heterogeneous IoT gateways, balancing performance, latency, and energy considerations [12].
Partial offloading techniques have also gained traction, particularly in AI inference. Zhang et al. presented a partitioning mechanism whereby only computationally heavy layers of a neural network are offloaded to specialized infrastructure, while simpler layers run on the edge device [13]. Hassan et al. and Moreno et al. examined lightweight containerization at the edge, demonstrating that resource overhead can be minimized through optimized container runtimes such as Docker, containerd, and CRI-O [14][15].
Study | Contribution | Findings | Implications |
---|---|---|---|
[16] Chiang et al. | Proposed an edge–fog–cloud migration framework | Demonstrated dynamic workload relocation based on resource availability | Highlighted potential for reduced overall carbon footprint |
[18] Qiu et al. | Developed sleep-mode protocols for 5G base stations | Showed drastic energy reduction during off-peak usage | Enabled significant cost savings and lowered emissions |
[17] Yang et al. | Introduced carbon-intensity-aware scheduling | Aligned workload placement with regional grid data | Improved sustainability in multi-tier edge–fog–cloud environments |
[22] White et al. | Proposed standardized carbon footprint metrics | Offered a uniform reporting structure for edge infrastructures | Facilitated consistent policy and regulatory compliance |
[24] Johnson et al. | Analyzed economic incentives for green edge computing | Demonstrated effectiveness of tax benefits and carbon credits | Encouraged broader adoption of low-power deployments |
System-Level Coordination and Policy Frameworks
A holistic perspective that spans hardware, network, and orchestration layers has been pivotal in advancing carbon footprint reduction. Chiang et al. and Yang and Li introduced integrated edge–fog–cloud architectures, showing how workload migration across geographically distributed nodes can leverage variations in carbon intensity [16][17]. Qiu et al. and Nguyen et al. developed adaptive networking protocols to reduce base-station energy consumption, such as utilizing sleep modes during off-peak periods or coordinating workload consolidation across neighboring edge gateways [18][19]. These system-wide efforts are increasingly driven by AI-based methods, where machine learning algorithms predict resource utilization or carbon intensity to trigger proactive power management [20][21].
Policy and regulation also play a crucial role. White et al. underscored the need for standardized carbon footprint metrics in edge infrastructures [22], while Gao et al. examined regional regulations enforcing minimum energy efficiency levels for gateways and micro data centers [23]. Johnson et al. explored how carbon credits or tax benefits can incentivize low-power chipset adoption, and Schaefer et al. investigated the impact of green certifications on consumer purchasing behaviors [24][25]. Devic et al. integrated eco-design principles, such as modular battery packs and real-time energy monitoring, to extend hardware life and reduce e-waste [26].
Key Strategies for Reducing Carbon Emissions
Recent publications demonstrate that strategies to mitigate carbon emissions in edge computing frequently span multiple system layers. Hardware-centric measures include deploying ultra-low-power SoCs, optimizing chip layouts, and adopting novel packaging materials to improve heat dissipation. Complementary software-based techniques revolve around power-aware scheduling, partial offloading, and containerized orchestration with minimal resource overhead. AI-driven coordination has also gained traction in predicting workload spikes, carbon intensity variations, and thermal thresholds, thus enabling proactive resource scaling.
Integrating localized renewable energy sources such as solar or wind power at edge sites can enhance sustainability, although practical deployment remains challenging in certain regions. Government policies and industry standards further encourage the adoption of green practices, including energy efficiency mandates and carbon credits. Eco-design principles, which consider recyclability and modular maintenance, help to reduce e-waste and extend device lifespans.
Dimension | Techniques / References | Contributions | Findings |
---|---|---|---|
Hardware | Low-power SoCs ([4] Xu et al.) and AI accelerators ([6] Ramesh et al.) | Minimized idle power and specialized hardware for inference | Notable reductions in power usage across diverse workloads |
Software | DVFS with reinforcement learning ([11] Martinez et al.) and partial offloading ([13] Zhang et al.) | Dynamically adjusted CPU frequency and partitioned compute tasks | Demonstrated adaptive energy savings under varying load conditions |
System Orchestration | Edge–fog–cloud migration ([16] Chiang et al.) and container optimization ([14] Hassan et al.) | Relocated tasks across network layers using lightweight virtualization | Improved resource utilization and reduced operational overhead |
Policy/Regulation | Carbon credits ([24] Johnson et al.) and standardized metrics ([22] White et al.) | Encouraged greener practices through financial and reporting mechanisms | Facilitated consistent adoption of sustainability measures across stakeholders |
Open Challenges
Despite clear progress, several open challenges persist. One concern is the wide heterogeneity of edge devices, complicating unified energy-saving approaches. Energy monitoring and carbon-intensity data are not consistently available worldwide, impeding real-time or dynamic optimizations [17]. Trade-offs between reliability and energy efficiency are particularly evident in mission-critical scenarios such as autonomous vehicles or healthcare, where service disruptions or latency spikes may be unacceptable [27]. Current policy frameworks differ across regions, creating fragmented regulations and disjointed compliance requirements for global operators [23]. Furthermore, security and privacy concerns arise when implementing AI-driven power management and data collection, as such systems may become attack vectors or inadvertently compromise sensitive user information [21].
Future Directions
Federated learning for energy management represents a promising avenue, allowing distributed edge nodes to collaborate on model training without consolidating sensitive data [21]. Cross-layer co-design, integrating hardware, operating system functionality, and application-level optimizations, could offer more substantial efficiency gains than focusing on single layers. The development of dynamic carbon-aware energy markets, where edge nodes can schedule tasks based on real-time prices and carbon intensity, also presents a compelling framework for sustainable resource allocation [17]. Standardized metrics and benchmarking tools for energy usage and emissions, analogous to data center metrics like Power Usage Effectiveness (PUE), would further facilitate solution comparisons across device types and vendors, while life-cycle assessments (LCAs) need to be embedded into procurement processes for edge hardware [28].
References
- Shi, Weisong (2020). "Edge computing: Vision and challenges". IEEE Internet of Things Journal, 7(5): 4238–4260.
- Satyanarayanan, Mahadev (2019). "The Emergence of Edge Computing". Computer, 52(8): 30–39.
- Abdollahi, Ali (2019). "Environmental implications of micro data centers: A case study". Sustainability, 11(10): 2728.
- Xu, Lili (2019). "Energy-efficient SoC design for IoT edge devices". IEEE Transactions on Circuits and Systems I: Regular Papers, 66(8): 2952–2963.
- Mendez, Carlos (2020). "Heterogeneous multicore edge processors for power-aware embedded systems". ACM Transactions on Design Automation of Electronic Systems, 25(6): Article 40.
- Ramesh, K. (2022). "Low-power AI accelerators for on-device computer vision". IEEE Transactions on Circuits and Systems for Video Technology, 32(2): 360–372.
- Bae, Seunghyun (2021). "Sustainable manufacturing of edge devices: A holistic analysis". Journal of Manufacturing Systems, 60: 426–439.
- Kim, Hyunsu (2023). "Biologically inspired materials for enhanced cooling in edge devices". Nature Electronics, 6(2): 153–164.
- Liu, Qian (2019). "Liquid cooling in micro data centers at the edge: A quantitative study". IEEE Transactions on Industrial Informatics, 15(10): 5551–5561.
- Wan, Jiafu (2018). "Dynamic voltage and frequency scaling for real-time systems in edge computing". Journal of Parallel and Distributed Computing, 119: 29–40.
- Martinez, Daniel (2021). "Reinforcement learning-based DVFS control for energy-efficient edge nodes". IEEE Transactions on Sustainable Computing, 6(3): 403–414.
- Li, Guanyu (2019). "Multi-objective task scheduling for heterogeneous IoT gateways in edge computing". Future Generation Computer Systems, 100: 223–238.
- Zhang, Fan (2022). "Partial offloading of convolutional neural networks in edge computing environments". IEEE Transactions on Industrial Electronics, 69(9): 8957–8967.
- Hassan, Ahmed (2021). "Performance and energy analysis of containerization in edge micro data centers". IEEE Access, 9: 79028–79039.
- Moreno, Juan (2023). "Kubernetes-based energy-aware orchestration for edge computing". ACM Transactions on Internet Technology, 23(1): Article 5.
- Chiang, Mung (2018). "Fog and IoT: An overview of research opportunities". IEEE Internet of Things Journal, 5(4): 2451–2461.
- Yang, Yifan (2023). "Carbon-intensity-aware workload placement in hybrid edge-fog-cloud environments". IEEE Transactions on Cloud Computing, 11(2): 548–561.
- Qiu, Xiaobo (2020). "Adaptive sleep-mode protocols for 5G base stations in edge scenarios". IEEE Transactions on Green Communications and Networking, 4(3): 670–683.
- Nguyen, Tuan (2021). "Collaborative workload consolidation for green edge computing". IEEE Transactions on Sustainable Computing, 6(4): 998–1009.
- Tang, Wei (2019). "AI-driven power management in IoT edge devices using deep Q-networks". Sensors, 19(18): 4043.
- He, Sheng (2022). "Federated learning for energy efficiency in edge networks: A novel predictive approach". Computer Networks, 202: 108614.
- White, Robert (2020). "Standardizing carbon footprint metrics in edge computing infrastructures". IEEE Communications Standards Magazine, 4(3): 12–19.
- Gao, Yuan (2023). "Regional regulations and compliance for edge energy efficiency: Framework and insights". IEEE Transactions on Sustainable Computing, 8(2): 546–560.
- Johnson, Tyler (2021). "Incentivizing low-power edge deployments through carbon credits and tax reductions". IEEE Transactions on Industrial Informatics, 17(9): 6402–6413.
- Schaefer, Vanessa (2022). "Green labels for IoT devices: Consumer awareness and adoption". Electronic Markets, 32(3): 1041–1056.
- Devic, Aleksandar (2024). "Eco-design in edge hardware: Modular battery packs and real-time energy monitoring". IEEE Transactions on Components, Packaging and Manufacturing Technology, 14(3): 312–324.
- Sakr, Mahmoud (2020). "A game-theoretic approach to minimizing energy consumption via offloading in edge-cloud environments". IEEE Transactions on Mobile Computing, 19(11): 2624–2638.
- Du, Shengnan (2019). "Life cycle analysis of IoT devices: Energy, emissions, and disposal". Journal of Cleaner Production, 231: 341–350.
7.5 Data Persistence
7.5.1 Introduction
What is Edge Persistence?
Data persistence plays a crucial role in ensuring that data generated is reliably stored and managed locally or near the location, even in volatile environments. Persistence at the edge is especially important, as edge applications are often deployed in highly non-deterministic conditions. By bringing data closer to the edge, it becomes essential to ensure its reliability. Exploring persistent storage allows us to safeguard data integrity by capturing information locally until it can be properly synchronized. Edge persistence ensures that data remains accessible, secure, and consistent even in network disruptions, hardware failures, or resource constraints, which is essential for maintaining reliability in edge computing environments [8].
This section introduces the topic of persistence at the edge highlighting prominent challenges in the space of data integrity in distributed edge systems. This section explores three main challenges of data persistence at the edge:
- Byzantine Faults
- Data Consistency
- Limited Resources
This section will also explore emerging solutions to these challenges that are not standardized or concrete, but serves as a direction in which to consolidate these answers to solve data integrity at the edge:
- Store-and-Forward Techniques: using message queues (MQTT or Apache Kafka) to reliably forward data when the connection resumes [6].
- Lightweight Databases: Use embedded databases optimized for low-resource environments (e.g., SQLite, TinyDB, or RocksDB).
- Conflict Resolution Policies: Use mechanisms like versioning, timestamps, or last-write-wins to resolve conflicting updates [5].
7.5.2 Architectural Models for Edge Storage
Overview
The primary goal of edge computing is to shift data processing closer to the edge, in doing so it reduces latency. Although this decentralized approach also brings challenges on how data is stored. Architectural models for edge persistence must balance [1] :
- performance
- scalability
- consistency
Centralized Storages
In a centralized model edge nodes collect data and are sent to a central node. This model is prominent in cloud computing which simplifies data management, but also introduces latency and single point of failure [2]. Centralization offers maintainability and control over data policies. It also is subjected to vulnerabilities in network failures[3].
Decentralized Storages
Decentralized storage involves storing and processing data in edge devices across a distributed system of nodes. This model offers fault tolerance and a reduction in latency, but a higher complexity of data management[4]. It offers better support for real-time applications, but a more complex model in need of more data synchronizations and data consistency[5].
Hierarchical Storage Architectures
Hierarchical models organize storage across tiers:
- Tier 1 (Device Level): Sensors, gateways, or IoT devices store small amounts of critical data locally.
- Tier 2 (Edge Nodes): More capable edge servers store larger volumes, perform processing, and temporarily cache data.
- Tier 3 (Cloud/Core): Central data centers serve as long-term storage and coordination points16. This tiered approach helps balance performance and cost while offering flexibility in data handling.
Peer-to-Peer and Mesh-Based Storage
Peer-to-peer (P2P) or mesh-based architectures involve edge nodes communicating directly to share and replicate data. These systems are used in scenarios with unreliable connectivity, employing techniques like gossip protocols, data sharding, and replication to maintain availability and redundancy[6].
7.5.3 Persistence Mechanisms in Edge Devices
Caching Strategies
Caching is a critical persistence mechanism in edge computing, as it accelerates data access by storing frequently accessed data closer to the point of use. Techniques like in-memory caching, distributed caching, and content delivery networks (CDNs) are commonly used to enhance performance and reduce latency. For instance, in-memory caching stores data directly within an application's memory space, offering the fastest access times due to its proximity to the application logic.
Local Databases
Local databases are essential for storing and managing data on edge devices. SQLite is a lightweight relational database ideal for embedded systems, mobile apps, and IoT devices due to its small footprint and ease of use. On the other hand, RocksDB is a high-performance key-value store optimized for write-heavy workloads and large-scale applications, making it suitable for edge AI solutions and real-time analytics.
Object Storage at the Edge
Object storage is increasingly used at the edge due to its scalability and flexibility. It simplifies operations by avoiding hierarchical file systems and supports peer-to-peer architectures, which enhance flexibility and efficiency across distributed computing environments. Edge object storage is particularly beneficial for handling fluctuating workloads and accommodating modern technologies like containerization and microservices.
7.5.4 Synchronization and Data Consistency
Data Consistency at the edge
Data consistency is an integral component of Persistence at the edge. This subcomponent refers to ensuring that data remains consistent and accurate in a distributed system where data homogeneity remains firm. The challenge of maintain data consistency is prominent because edge devices are not resilient to environmental resource scarcity, which often leads to network issues or hardware failures. Challenges of consistency include: connectivity, latency, and data integrity. A way to solve these issues are consistency protocols. [3]
CRDT (Conflict-Free Replicated Data Types
CRDTs are data structures implemented in distributed systems that allow modification independently at multiple locations, while also ensuring changes are merged without any conflicts. CRDTs are useful in edge environements where multiple replicase of data may exist and must be synchronized in local caches, or CDNs. CRDTs follow three main components:
- Conflict Free
- Replication
- Consistency
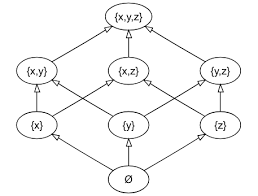
7.5.5 Latency and Throughput Considerations
7.5.6 Fault Tolerance and Recovery
Byzantine Faults at the Edge
Byzantine faults refer to an issue where in some distributed system, nodes (devices) may behave un predictably due to failures or attacks. Faulty nodes may send incorrect data which results in data corruption that affects other nodes in the server. This problem is essential to explore in edge systems as one main challenge of edge computing is the unreliability of resource constraint devices. Devices located to data sources tend to be highly unpredictable (e.g. IoT devices, mobile phones, or embedded systems). There are many algorithmic solutions to reduce Byzantine faults such as Bracha-Toueg Byzantine consensus algorithm, Mahaney-Schneider synchronizer, and other consensus algorithms,
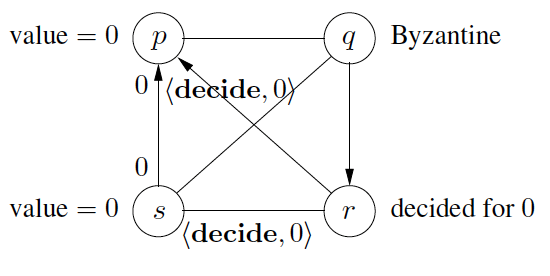
Byzantine Generals Problem, which was introduced by Leslie Lamport, Robert Shostak, and Marshall Pease in their 1982 paper titled The Byzantine Generals Problem
Case Study 1: Byzantine resilience in federate learning at the edge
Creative solutions in edge systems are explored in [1], Byzantine resilience in federated learning at the edge, where heavy tailed data and communication inefficiencies are main challenges presented in this paper. Federated learning is a prominent example of a edge tailored system where Byzantine resilience is essential. Authors of this paper proposed a distributed gradient descent algorithm that operates with local computations on each device where it calculates the gradient of the model based on local data. In order to trim failed nodes, the algorithm proposed uses coordinate-wise trimmed mean. This algorithm is designed to follow heavy tailed distribution which ensures outliers do not affect the model. The key issues addressed in the paper is how Byzantine failures degrade the performance of federated learning systems. This solution employes robust aggregation in order to solve these issues where traditional algorithms are inapplicable.
7.5.7 Edge Persistence in Modern Frameworks
Integration with Kubernetes and K3s
Leveraging Persistent Volumes in Edge Clusters
Edge DBs and Lightweight Orchestration Tools
7.5.8 Conclusion
References
- arXiv, "Defining a Reference Architecture for Edge Systems in Highly Uncertain Environments," arXiv, 2024, [Online]. Available: https://arxiv.org/pdf/2406.08583.pdf.
- G. Jing, Y. Zou, D. Yu, C. Luo and X. Cheng, "Efficient Fault-Tolerant Consensus for Collaborative Services in Edge Computing," in IEEE Transactions on Computers, vol. 72, no. 8, pp. 2139-2150, 1 Aug. 2023, doi: 10.1109/TC.2023.3238138.
- Y. Tao et al., "Byzantine-Resilient Federated Learning at Edge," in IEEE Transactions on Computers, vol. 72, no. 9, pp. 2600-2614, 1 Sept. 2023, doi: 10.1109/TC.2023.3257510.
- G. Cui et al., "Efficient Verification of Edge Data Integrity in Edge Computing Environment," in IEEE Transactions on Services Computing, vol. 15, no. 6, pp. 3233-3244, 1 Nov.-Dec. 2022, doi: 10.1109/TSC.2021.3090173.
- Y. Lin, B. Kemme, M. Patino-Martinez and R. Jimenez-Peris, "Enhancing Edge Computing with Database Replication," 2007 26th IEEE International Symposium on Reliable Distributed Systems (SRDS 2007), Beijing, China, 2007, pp. 45-54, doi: 10.1109/SRDS.2007.10.
- E. Chandra and A. I. Kistijantoro, "Database development supporting offline update using CRDT: (Conflict-free replicated data types)," 2017 International Conference on Advanced Informatics, Concepts, Theory, and Applications (ICAICTA), Denpasar, Indonesia, 2017, pp. 1-6, doi: 10.1109/ICAICTA.2017.8090961.
- S. SM, A. Bhadauria, K. Nandy and S. Upadhyay, "Lightweight Data Storage and Caching Solution for MQTT Broker on Edge - A Case Study with SQLite and Redis," 2024 IEEE 21st International Conference on Software Architecture Companion (ICSA-C), Hyderabad, India, 2024, pp. 368-372, doi: 10.1109/ICSA-C63560.2024.00066.
- DataBank, "Securing the Edge: Addressing Edge Computing Security Challenges," DataBank Blog, [Online]. Available: https://www.databank.com/resources/blogs/securing-the-edge-addressing-edge-computing-security-challenges/ (Accessed: April 8, 2025)
- Explo, "Top 8 Embedded SQL Databases in 2025," Explo Blog, 2025, [Online]. Available: https://www.explo.co/blog/embedded-sql-databases.
- TechTarget, "How edge object storage aids distributed computing," TechTarget, 2021, [Online]. Available: https://www.techtarget.com/searchstorage/feature/How-edge-object-storage-aids-distributed-computing.
- Dong et al., "Evolution of Development Priorities in Key-value Stores Serving Large-Scale Applications," FAST '21, 2021, [Online]. Available: https://www.usenix.org/system/files/fast21-dong.pdf.